Guide: in order to solve the problems of heterogeneous data source access, slow I/O speed of storage and calculation separation, low efficiency of scenario perception and low scheduling, the open source project fluid was jointly launched by pasalab, Alibaba and alluxio of Nanjing University in june2020 in order to solve the problems of heterogeneous data source access, slow storage / computing separation I/O speed, low efficiency of scenario perception and scheduling.
Fluid is an efficient support platform for data intensive applications in cloud native environment. Since the open source release, the project has attracted the attention of experts and engineers in many related fields. The community has been evolving with the positive feedback of all. Recently, fluid 0.5 version has been officially released. In this version, fluid mainly adds three aspects to improve:
-
It is rich in the operation functions of data sets, and supports online elastic expansion, metadata backup and recovery.
-
Support the deployment of various environments and meet the user's personalized deployment and configuration requirements.
-
Add data cache engine implementation, and increase the engine selection of users on the public cloud.
Fluid open source project address : https://github.com/fluid-cloudnative/fluid
The development requirements of these three main functions come from the actual production feedback of many community users. In addition, fluid v0.5 has also carried out some bug fixes and document updates. Welcome to experience fluid v0.5!
Fluidv0.5 download link : https://github.com/fluid-cloudnative/fluid/releases
The following is a further introduction to the release features of this new version.
Enrich the operation function of data setโ
In this version, fluid focuses on enriching the relevant operation functions of the core abstract object, dataset, so that data intensive applications can better utilize the basic functions of elasticity and observability provided by cloud natively, and enhance the user's flexibility in data set management.
1. data set online elastic cache expansionโ
This is the function that community users have been looking forward to! Before fluid v0.5, if the user wants to adjust the cache capability of the dataset, it needs to be done by uninstalling the cache engine and redeploying it all. This approach is time-consuming and must also consider the high cost of all data cache loss. Therefore, in the new version, we provide the support for the data set to expand the cache elasticity. Users can increase the cache capacity of a dataset on-the-fly in a non-stop manner according to their own scenario requirements to accelerate data access (expansion) or reduce the cache capacity (shrink) of a dataset that is not frequently used, Thus, more precise elastic resource allocation can be realized and resource utilization rate can be improved. The built-in controller of fluid selects the appropriate expansion node according to the policy, for example, when scaling, it will take the task situation on the node and the node cache ratio as the filter condition.
To perform the elastic data set cache capacity elastic expansion, the user only needs to run the following command:
kubectl scale alluxioruntimes.data.fluid.io {datasetName} --replicas={num}
Where dataset name corresponds to the name of the dataset, replica specifies the number of cache nodes.
The video of manual expansion and its effect of data set is as follows:
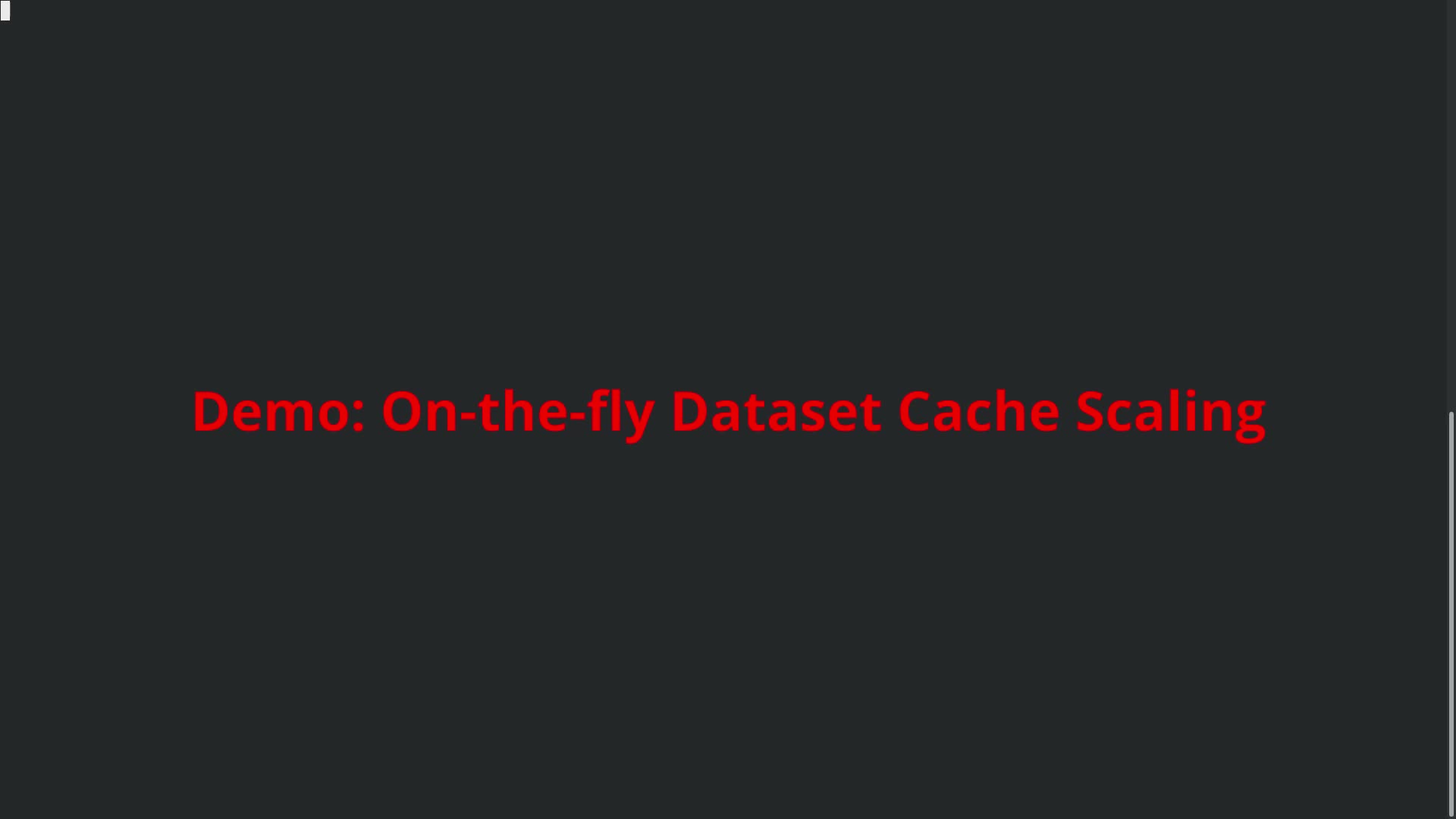
For more details on manual scaling of datasets, refer to documentation
This feature enhances the flexibility of fluid dataset metadata management. Previous fluid v0.4 has supported loading metadata for datasets (for example, file system inode tree) to the local and records some key statistics (for example, the size of the data volume and the number of files) of the dataset. However, once the user destroys the local dataset, all the metadata information will be lost, and the data set needs to be retrieved from the underlying storage system again when rebuilding the dataset.
Therefore, in fluid v0.5, we add a k8s custom resource object, DataBackup, which provides the user with a declarative API interface to control the related behavior of data backup. A simple example of building a DataBackup custom resource object is as follows:
apiVersion: data.fluid.io/v1alpha1
kind: DataBackup
metadata:
name: hbase-backup
spec:
dataset: hbase
backupPath: pvc://<pvcName>/subpath1/subpath2/
When you create the dataset again, you need to add a field that specifies the location of the backup file:
apiVersion: data.fluid.io/v1alpha1
kind: Dataset
metadata:
name: hbase
spec:
dataRestoreLocation:
path: pvc://pvc-local/subpath1/
mounts:
- mountPoint: https://mirrors.tuna.tsinghua.edu.cn/apache/hbase/2.2.6/
At this point, fluid will first load metadata and dataset statistics from the backup file, thus greatly improving the loading speed of metadata.
3. observability optimization of data setโ
Fluid v0.5 also further enhances the observability of the dataset, which includes two parts:
1) combination with Prometheusโ
This feature supports the collection of data set availability and performance indicators and is visualized through grafana. At present, the implementation of alloxioruntime is supported. Users can easily understand the performance indicators such as current cache node, cache space, existing cache ratio, remote reading, short-circuit reading and so on. The whole configuration process is very simple, and it achieves the effect of "out of the box" for the data set monitoring system.
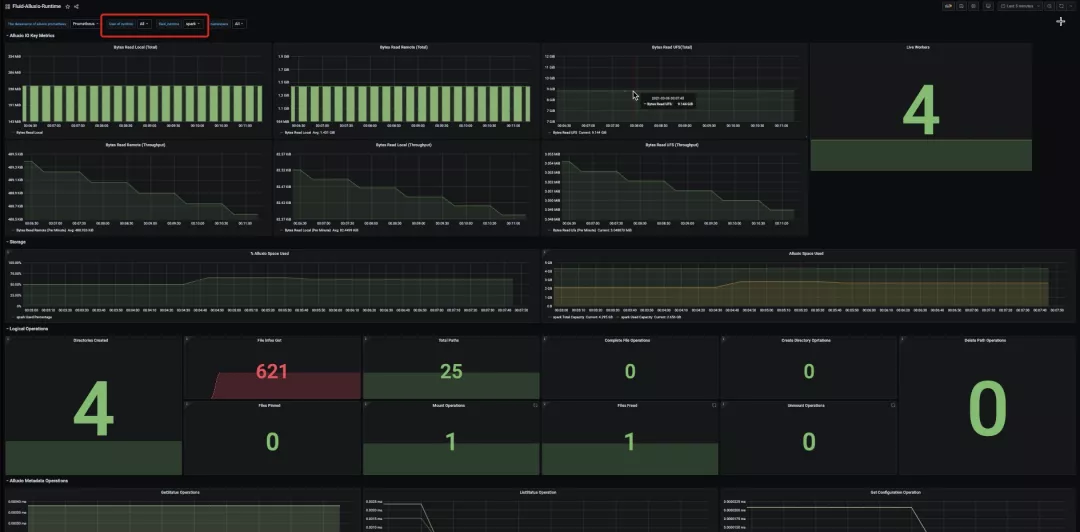
2) hit rate index of new dataset cacheโ
This feature can identify how many of the access to the dataset in the last 1 minute has hit the distributed cache. On the one hand, the indicator can help users analyze the performance bottleneck in their data intensive applications, and quantitatively check the effect of fluid in the workflow of the whole application; On the other hand, it can help users to balance the application performance and cache resource occupation, and make reasonable expansion decision.
This indicator is added to the dataset CRD resource status of 'dataset.status.cachestates' in the future v0.5, specifically including:
-
Cache hit ratio: the percentage of access to distributed cache hits in the past minute.
-
Local hit ratio: the percentage of access hit by the local cache in the past minute.
-
Remote hit ratio: the percentage of access to remote cache hits in the past minute.
Note: for distributed cache, there are two different cache hits for data hits Local cache hit refers to the access initiator can access the cache data directly at the same node Remote cache hit refers to the access to cache data on other nodes through the network by the initiator.
In fluid v0.5, users can easily view cache hit indicators using the following command:
kubectl get dataset <dataset-name> -o wide
NAME ... CACHE HIT RATIO AGE
<dataset-name> ... 86.2% 16m
Support deployment of diverse environment configurationโ
Since the release of fluid 0.4, we have increased support for fluid deployment configuration in a variety of environments according to the problems and requirements of community users' actual deployment feedback.
1. support fuse global modeโ
In fluid, the remote files defined in the dataset resource object are schedulable, which means that you can manage the remote file cache to the location on the kubernetes cluster as you do managing pod. The calculated pod can access the data file through the fuse client. In previous versions of fluid, fuse clients always schedule to the nodes where the cache is located, but users are not free to control the dispatch of fuse.
In fluid v0.5, we added a global deployment pattern to fuse. In this mode, fuse is deployed globally to all nodes by default. Users can also influence the scheduling results of fuse by specifying the nodeselector of fuse. At the same time, cache will be deployed on nodes with a large number of calculated pods.
2. support HDFS user level configurationโ
Many community users use the distributed cache system, alloxo, as the cache engine for fluid data sets. In the case of data set persistence stored in HDFS file system, to make aluxo access to the underlying HDFS, the aluxo cluster needs to obtain all kinds of configuration information of the HDFS in advance.
In fluid v0.5, we use kubernetes' native resources to support the above scenarios. Users need to create the relevant configuration files (e.g. ` HDFS site.xml 'and' core site.xml ') in the kubernetes environment in the form of' configmap ', and then reference the' configmap 'created above in the created' alloxioruntime 'resource object to achieve the above functions.
An example of the alloxioruntime
resource object is as follows:
apiVersion: data.fluid.io/v1alpha1
kind: AlluxioRuntime
metadata:
name: my-hdfs
spec:
...
hadoopConfig: <configmap-name>
...
At this point, the created cluster of aluxo will be able to access the data in the HDFS cluster normally. For more information, refer to the sample documentation
Implementation of new data cache engineโ
The default distributed cache runtime used by fluid is alluxioruntime. In order to support the needs of users in different environments for the cache system, fluid has made the distributed cache runtime access framework into a pluggable architecture in the previous version. In fluid v0.5, community contributors from Alibaba cloud developed jindoruntime based on the framework and added an execution engine implementation to support fluid dataset data management and caching. Users can use the cache mode of jindofs to access and cache remote files in fluid through jindoruntime. Using and deploying jindoruntime on fluid is simple, compatible with the native k8s environment and out of the box.
Summaryโ
In fluid v0.5, we have enriched and enhanced the functional features and user experience of fluid.
First of all , fluid v0.5 further adds the function operation of dataset:
-
Provide online elastic capacity expansion and contraction of data sets, and realize more flexible and fine cluster resource allocation control.
-
The new DataBackup CRD realizes the backup and recovery of dataset file metadata and other information, and helps complete the rapid restart of dataset caching system.
-
A cache hit rate indicator is added to help users better quantify and analyze the acceleration effect provided by fluid.
Secondly , fluid supports more environment modes and configurations to meet the deployment requirements of more real scenarios.
Finally , fluid adds a distributed cache runtime based on jindofs - jindoruntime, which provides users with different cache engine choices in diversified deployment environments.
We will continue to pay extensive attention to and adopt community suggestions to promote the long-term development of the fluid project, and look forward to hearing more feedback from you. If you have any questions or suggestions, welcome to join the fluid user group to participate in communication or discuss with us on GitHub:
Acknowledgeโ
Thanks to the community partners who contributed to this version, including Wang Tao from Alibaba cloud, Xie Yuandong from Tencent cloud, Qiu Lingwei from China Telecom, Xu Zhihao, Hou Haojun, Chen Guowang, Chen Yuquan and other students from pasalab of Nanjing University.
Introduction to the authorโ
Dr. Gu Rong, associate researcher of Computer Department of Nanjing University, member of PMC of fluid open source project co founder and alluxio open source project, research direction of big data processing system, has published more than 30 papers in Frontier Journal conferences in TPDS, ICDE, jpdc, IPDPS, ICPP and other fields, and presided over general projects / youth projects of National Natural Science Foundation of China There are a number of projects specially funded by China Postdoctoral Science Foundation. The research results have been applied to Alibaba, Baidu, byte beat, Sinopec, Huatai Securities and other companies and open source projects Apache spark and alluxio, and won the first prize of Jiangsu Science and technology in 2018 and the youth science and technology award of Jiangsu computer society in 2019, Served as a member of the system software special committee of China Computer Society / communication member of the big data special committee and Secretary General of the big data special committee of Jiangsu computer society.